James Sharpnack, PhD (Duolingo)
Topic: AutoIRT: Improving the Fit of Item Response Theory Models Using Automated Machine Learning
High stakes tests rely on well-calibrated test item parameters, such as difficulty and discrimination. Accurately estimating item response theory (IRT) models from pilot data typically requires hundreds or even thousands of responses, depending on the complexity of the model. We show that we can use Automated Machine Learning (AutoML) to fit item response models based on item level features (e.g. word frequency) to speed up the piloting process and improve item parameter estimates. AutoML refers to tools, such as AutoGluon (http://auto.gluon.ai), that will automatically process raw data and provide accurate predictions without the need for significant fine-tuning, model selection, and manual experimentation. We develop a method called AutoIRT for fitting explanatory parametric item response functions (IRF) for dichotomous response data using item level features. AutoIRT contains 2 stages: a non-parametric explanatory model fits with AutoML, and a parametric model fit to the non-parametric IRF and fine-tuned to pilot data. We use this to fit 3 parameter logistic (3PL) models to response data for yes/no vocab items, where test takers are asked to identify if a given word is real or fake. To test the number of responses needed within a pilot we apply backtesting where we simulate a pilot in hindsight for ≈550 piloted items. We show that with AutoIRT, we can accurately estimate 3PL item parameters with 20 responses per item, when compared to fitting a non-explanatory 3PL model with 200 responses per item. We also show that we can provide “cold-start” IRT parameter estimates, i.e., with no pilot data, of reasonable quality. To fit our explanatory IRT model, we use linguistic features such as character n-gram frequencies in a corpus, CEFR level, and capitalization.
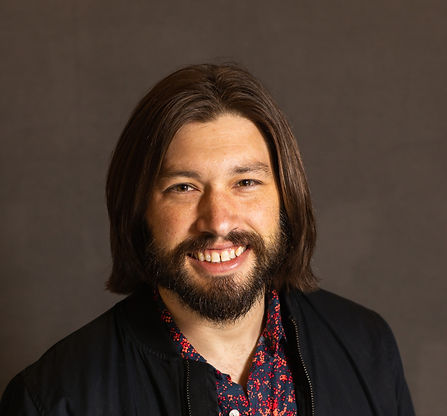
James received his B.S. from the Ohio State University in Mathematics and Physics, and his Ph.D. from Carnegie Mellon University in the joint program in Statistics and Machine Learning. He was a postdoctoral researcher in the Mathematics department at UC San Diego before he joined the Statistics department at UC Davis as an Assistant Professor. He became an Associate Professor in 2021, worked at Amazon Search as a Senior Applied Scientist, and has been at Duolingo as a Staff AI Research Scientist since 2022. His research lies at the intersection of statistics and machine learning, and he has over 50 published works in statistics journals, machine learning conferences, and scientific journals. This main area of research is in non-parametric statistics and machine learning and their applications to recommendation systems, epidemiology, astronomy, transportation science, and education. At Duolingo, he is developing Machine Learning powered test assessment for the Duolingo English Test, a high-stakes English language proficiency test.